As part of one of the
modules for my MSc in Remote Sensing and Planetary Science at Aberystwyth University, I did an assignment on land cover classification in Wales, using the LandSat satellites. I used data from Landsat 7 and Landsat 8.
The final maps from the assignment were somewhat basic, which I have revised below though I haven't changed the classification itself.
The classification scheme, was a rule-based one, where the rules were refined based on ground-control points taken using fieldwork.
I have put them into QGIS here, and added placename labels for context.
This is the first part of my revisiting this assignment. In future I will also say a little more about the ground control points from the fieldwork and how the results of the classification correspond to what was seen on the ground, and take this a little further than in the assignment report.
What would be really great, is to be able to automatically adjust a ruleset, rather than doing this by hand hard-coding into the scripts.
The Data
Landsat is a programme of Earth observation satellites launched by NASA and operated in cooperation with the US Geological Survey.
They have the capability to take data in several visible light bands, near infrared, short-wave infrared (this is longer wavelength than near-infrared but the common terminology in Earth Observation), and thermal infrared.
Landsat 8 has a slightly different range of wavelengths than Landsat 7, additionally having the 'Coastal' band in band 1 at a slightly shorter wavelength than the Blue band.
The area of study for the assignment was an area of mid-Wales including Aberystwth, and upland areas around Pumlumon.
We were set the images from Landsat 7 from March 2007 and June 2006 to work from, and I additionally used a Landsat 8 image from September 2013. Landsat 7 developer a
scan line corrector fault. which resulted in black stripes where no data was collected.
The black no data stripes were ignored, in this classification, my view was to classify based on the data that exists, and perhaps use a nearest neighbour interpolation right at the end after classification if desired.
The various Landsat bands allow an overview of land cover types, based on the differing reflectance spectral properties of vegetation of various types, water, and non-vegetated surfaces. Living vegetation is strongly reflectant in the green and near-infrared, with dead vegetation reflecting more strongly at the longer 'short-wave infrared'.
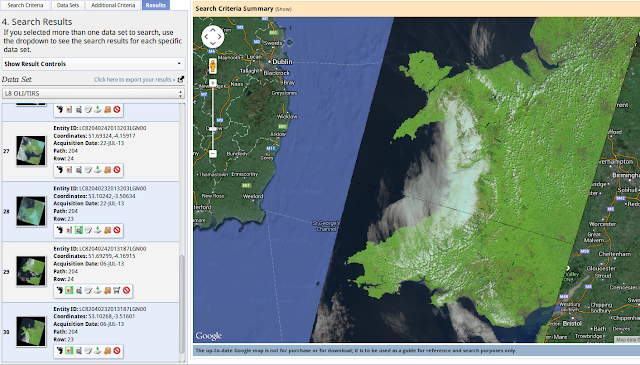 |
A bank of cloud coincides with the area of study on 6th July 2013 |
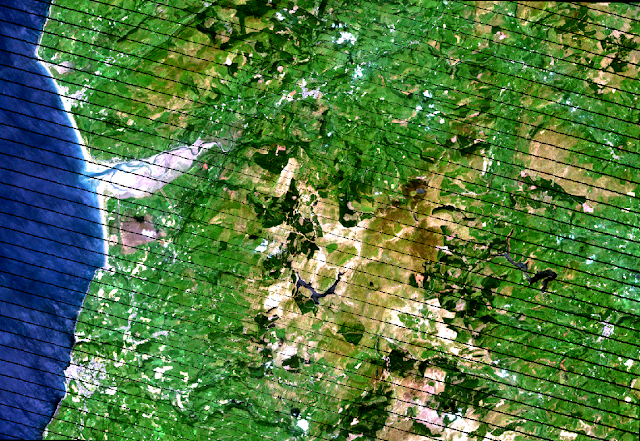 |
Landsat 7: 9th June 2006, using Bands 3, 2 and 1 (red, green and blue) as RGB. |
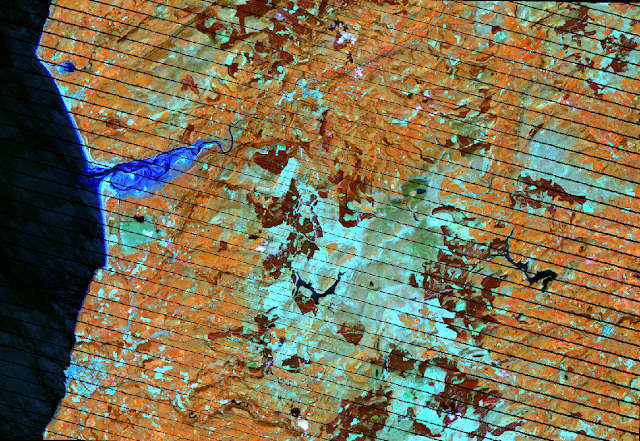 |
Landsat 7: 9th June 2006, using Bands 4, 6 and 3 (NIR, SWIR1 and red) as RGB. |
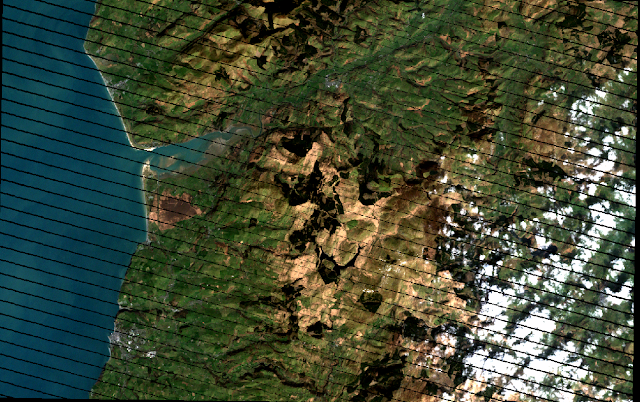 |
Landsat 7: 24th March 2007, using bands 3, 2 and 1 (red, green, blue) as RGB. |
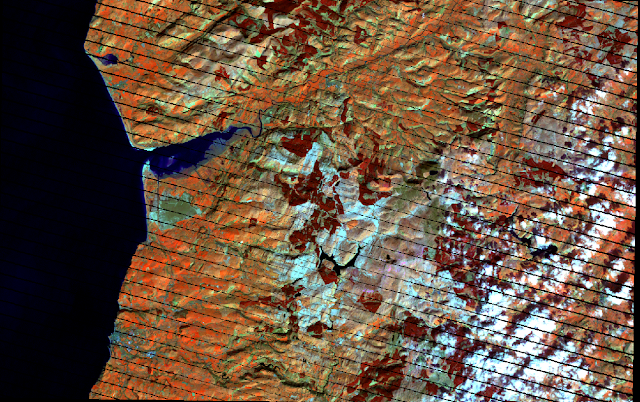 |
Landsat 7: 24th March 2007, using bands 4, 6 and 3 (NIR, SWIR1, red) as RGB. |
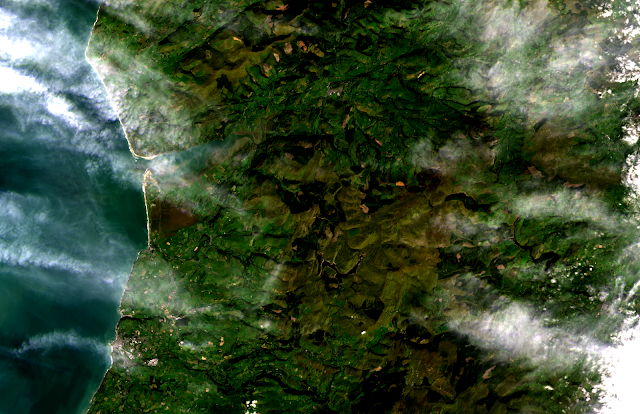 |
Landsat 8: 24th September 2013, bands 4, 3 and 2 (red, green, blue) as RGB. |
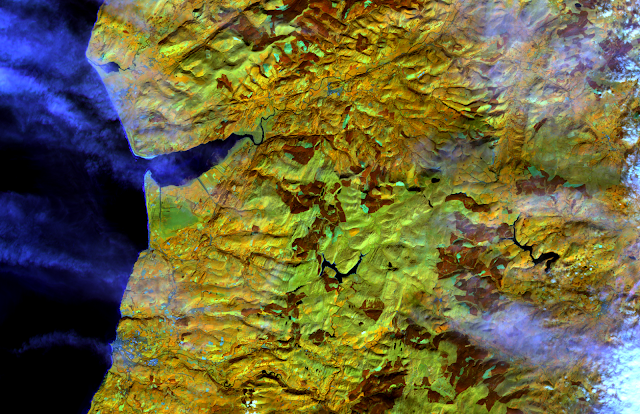 |
Landsat 8: 24th September 2013, bands 5, 6 and 4 (NIR, SWIR1, red) as RGB. |
Classification
A rule based classification was used, inspired broadly speaking by the various Richard Lucas et al. papers:
Lucas et al. 2007 Lucas et al. 2011.
Before classification, I first segmented to objects, for which I used the
routine in the Python RSGISLib libraries:
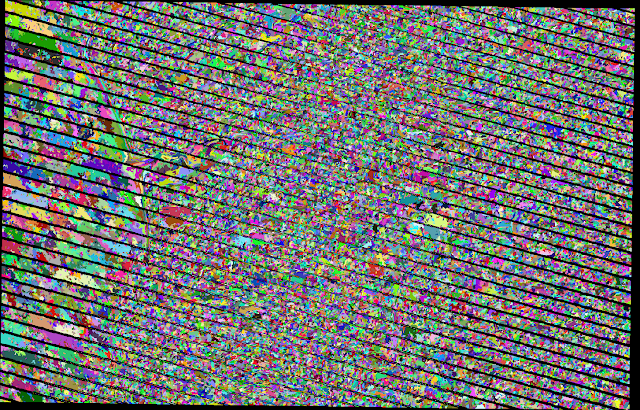 |
The 9th June 2006 image, segmented in RSGISLib using the runShepherdSegmentation method with 120 clusters and a minimum object size of 9 pixels (8100 sq.m), colourized randomly. |
In fact I segmented the image three different ways, in the assignment writeup I exclusively used the objects from segmenting the 24th March 2007 Landsat 7 image.
I made a kind of first order seasonal adjustment to the images, based on band averages in areas not classified as cloud or water. It was not entirely successful in creating a consistent classification as seen below.
Ruleset
The ruleset I first developed on the March 2007 image because that had areas of cloud and therefore an opportunity to get the cloud masking right first.
There are three stages to the process, first the Level 1A classification that delineates water (by low NIR and SWIR brightness), cloud (by high levels in the blue band), shadow (by thresholds in Blue, NIR, and SWIR1), and non-vegatation (by normalised differential vegatation index (NIR, R)).
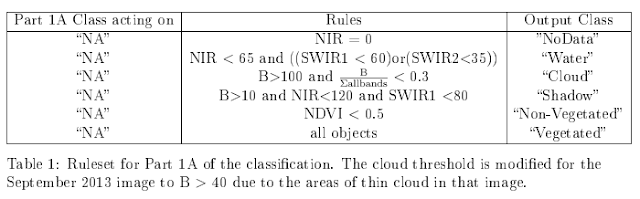
After this, in Level 1 split the vegetated areas into woodland, wetland, and heath, and grasslands into unimproved, semi-improved and improved by NDVI.
Level 2 classification splits woodland into broadleaf and coniferous, and wetlands into blanket bog and flush, and the upland vegetation further.
For the other images, I made a first-order seasonal adjustment based on the average band values in non-water and non-cloud objects, effectively attempting to adjust back to the March image. I adjust the NDVI by half of its actual change to avoid overcorrection of the grassland classes, and the Lucas et al. 2011 heath detection index by a manually set value of +3000 in June and +5000 in September.
24th March 2007 segmentation
This was what I used in my report. There is a substantial area under cloud in the SE of the image. I have masked out areas that have No Data in one or both images.
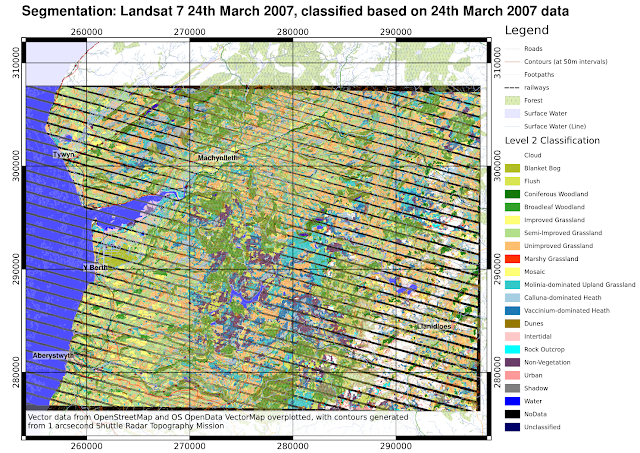 |
The cloud areas are shown in the SE, generally woodland and water extents are well-recovered. large version |
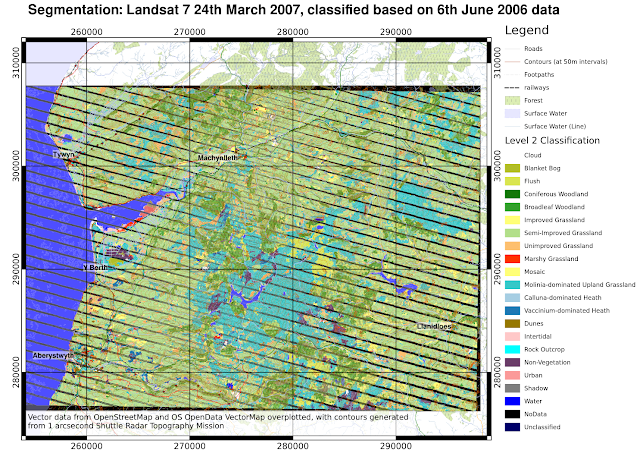 |
Unfortunately some detail is lost in the uplands, and some areas are spuriously classified as non-vegetated. large version |
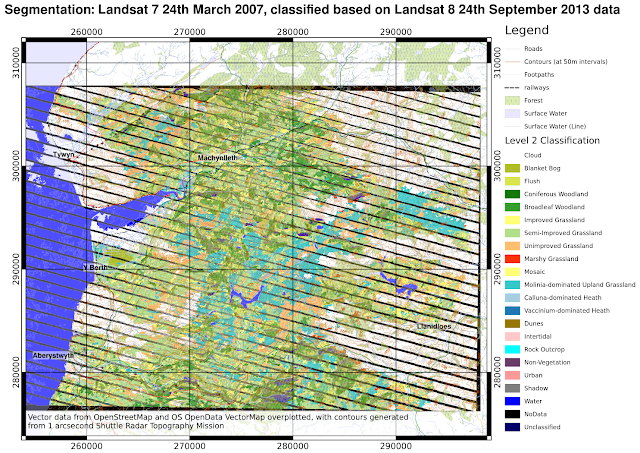 |
Modification of the cloud threshold was needed to mask out the extensive areas of thin cloud covering parts of the image. Some spurious water bodies are shown which are in face topographic shadow misclassified as water. large version |
6th June 2006 segmentation
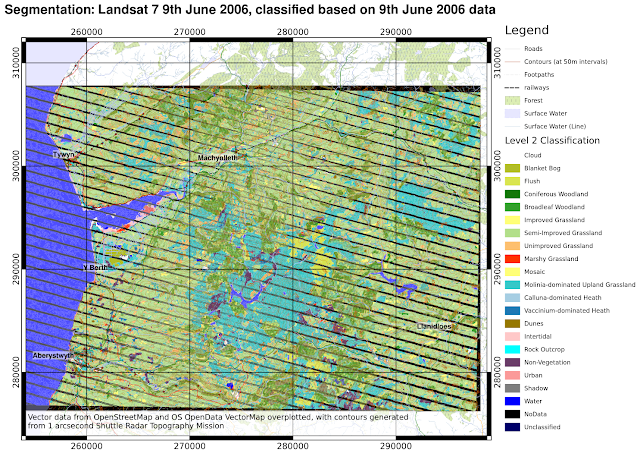 |
Again, the summer image does not differentiate the different type of upland vegetation well. The cloud shadow on Borth Bog is misclassified as water. large version |
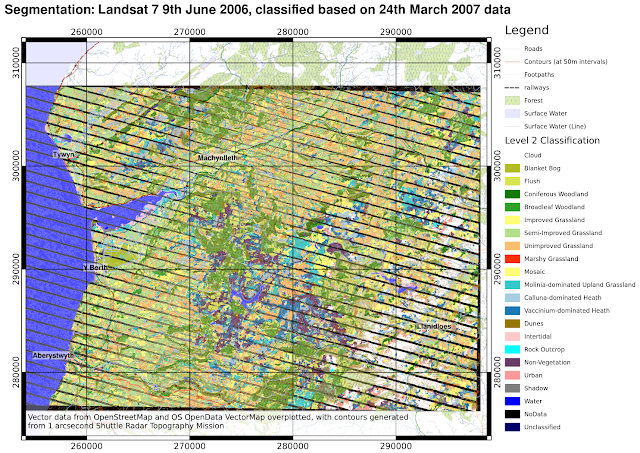 |
The summer 2006 image is segmented and the spring 2007 data applied, there are some spurious classifications in the cloud shadow areas. large version |
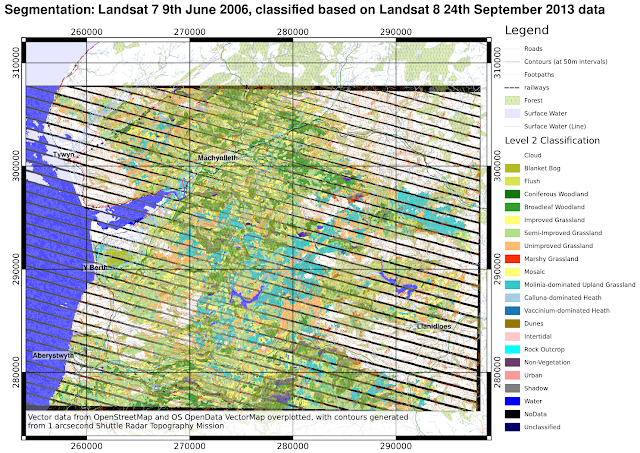 |
The September 2013 data, again showing some spurious areas of water, and some errors around the margins of cloud. large version |
24th September 2013 segmentation
I only present the 24th September 2013 data, for this segmentation, due to problems that would be caused by the Landsat 7 no data stripes.
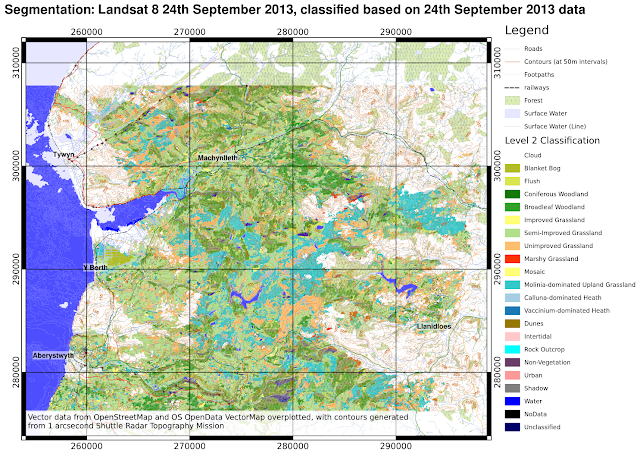 |
Using the Landsat 8 image for segmentation avoids the stripes of no data, but delimiting the edges of thin cloud is difficult and may result in spurious classifications around the edges. The upland vegetation is not well delineated, with large areas assigned to 'unimproved grassland' or 'Molinia-dominated upland grassland'. large version |